Rethinking Clustering for Robustness
Motasem Alfarra, Juan C. Pérez, Adel Bibi, Ali Thabet, Pablo Arbeláez, Bernard Ghanem
October 2021
Abstract
This paper studies how encouraging semantically-aligned features during deep neural network training can increase network robustness. Recent works observed that Adversarial Training leads to robust models, whose learnt features appear to correlate with human perception. Inspired by this connection from robustness to semantics, we study the complementary connection, from semantics to robustness. To do so, we provide a robustness certificate for distance-based classification models (clustering-based classifiers). Moreover, we show that this certificate is tight, and we leverage it to propose ClusTR (Clustering Training for Robustness), a clustering-based and adversary-free training framework to learn robust models.
Publication
In British Machine Vision Conference
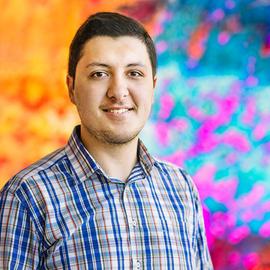
Machine Learning Researcher at Qualcomm AI Research, Amsterdam, Netherlands
I am a machine learning researcher at Qualcomm AI Research in Amsterdam, Netherlands. I obtained my Ph.D. in Electrical and Computer Engineering from KAUST in Saudi Arabia advised by Prof. Bernard Ghanem. I also obtained my M.Sc degree in Electrical Engineering from KAUST, and my undergraduate degree in Electrical Engineering from Kuwait University. I am interested in domain shifts, LLM safety, and how to combat them with test-time adaptation and continual learning. I helped co-organizing the first workshop on Test-Time Adaptation at CVPR2024!